Outlier-robust estimation of state-space models using a penalised approach
-
School of Mathematics and Statistics, University of Sydney, Sydney, Australia [rajan.shankar@sydney.edu.au, garth.tarr@sydney.edu.au]
-
Department of Quantitative Economics, Maastricht University, Maastricht, Netherlands [i.wilms@maastrichtuniversity.nl]
-
Department of Mathematics, University of Antwerp, Antwerp, Belgium [jakob.raymaekers@uantwerpen.be]
Keywords: state-space model – robustness – regularisation – animal tracking
1 Background
State-space models (SSMs) are a broad class of statistical models for modelling time-varying data. SSMs consist of an observed process (1) and a latent state process (2):
(1) | ||||
(2) |
where and are observational and state disturbances, is the state-transition matrix, and represents how states are transformed into observed measurements.
Practitioners use SSMs to infer the structure and dynamics of the state process based on the data from the observed process. However, when estimating state space models, it is common to assume that and follow normal distributions. This leads to intrinsically non-robust estimators which can result in poor parameter estimates in the presence of outliers.
There are techniques in the literature, such as that of Duran-Martin et al. [2024], to robustly estimate the states assuming that the SSM parameters — usually and the variances of and — are known. Our approach differs from these techniques by focusing on robustly estimating the SSM parameters as well.
2 Method
To robustly fit a SSM in the presence of outliers, we take inspiration from the iterative procedure for outlier detection (IPOD) developed by She and Owen [2011] who apply it to ordinary least squares regression models. They modify the regression model by adding extra parameters — one for each data point. If any of these parameters are estimated as non-zero, it suggests that the corresponding data point is an outlier. This formulation is known as a mean-shift formulation, and was earlier used by McCann and Welsch [2007]. We alter (1) to obtain a mean-shifted observed process:
where , , represent the shift parameters.
Since the model is over-parametrised, we impose sparsity among the ’s to prevent them from all being non-zero. This is achieved by using the hard penalty in the estimation procedure,
The tuning parameter controls how many data points are flagged as outliers, with smaller values of corresponding to more outliers. We use information criteria to choose an appropriate value of .
3 Results
We run a simulation study where we simulate data under the first-differenced correlated random walk (DCRW) model [Jonsen et al., 2005]; a type of state-space model commonly used for tracking the position of animals over time. We compare our robust method for fitting state-space models to classical approaches and the method of Crevits and Croux [2019]. Our method shows good performance in identifying outliers, as pictured in the example in Figure 1, and in parameter estimation.
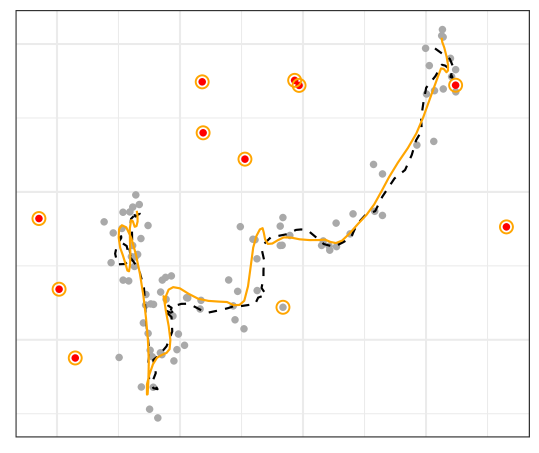
We also apply our method to animal-tracking data, such as polar bear and whale GPS measurements.
References
- Crevits and Croux [2019] Ruben Crevits and Christophe Croux. Robust estimation of linear state space models. Communications in Statistics - Simulation and Computation, 48(6):1694–1705, 2019.
- Duran-Martin et al. [2024] Gerardo Duran-Martin, Matias Altamirano, Alex Shestopaloff, Leandro Sánchez-Betancourt, Jeremias Knoblauch, Matt Jones, Francois-Xavier Briol, and Kevin Patrick Murphy. Outlier-robust Kalman filtering through generalised Bayes. In Proceedings of the 41st International Conference on Machine Learning, volume 235 of Proceedings of Machine Learning Research, pages 12138–12171, 21–27 Jul 2024.
- Jonsen et al. [2005] Ian D. Jonsen, Joanna Mills Flemming, and Ransom A. Myers. Robust state-space modeling of animal movement data. Ecology, 86(11):2874–2880, 2005.
- McCann and Welsch [2007] Lauren McCann and Roy E. Welsch. Robust variable selection using least angle regression and elemental set sampling. Computational Statistics & Data Analysis, 52(1):249–257, 2007.
- She and Owen [2011] Yiyuan She and Art B. Owen. Outlier detection using nonconvex penalized regression. Journal of the American Statistical Association, 106(494):626–639, 2011.