Primer on Data Science 2018
Trento, 27-29 June 2018
Aim
Primer on Data Science is a serie of summer schools organized by the curriculum Mathematics for Life and Data Sciences of the Laurea Magistrale in Mathematics (Department of Mathematics, University of Trento), to the aim of introducing third year bachelor students and bachelor graduates to the topics of this curriculum. Every year the school will have a different topic.
The 2018 edition will focus on a gentle introduction to some aspects of Bayesian Statistics and its applications in Epidemiology and Neural Activity.
Where
All the activities are in room A107 of Polo Scientifico e Tecnologico “Fabio Ferrari”, Povo 1, see here
Admission
The school is open to 30 participants, no fees are required, but registration is mandatory. Everybody is welcome to apply, however, admission will be based on the following criteria in order of importance
- Bachelor graduates and third year bachelor students in Mathematics
- Transcript of Records and grades
- Students from University of Trento
Lectures will be delivered in English.
Application is closed
Lectures
- Luigi Spezia (Biomathematics & Statistics Scotland, Bioss)
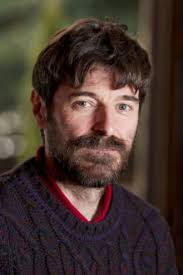
Luigi Spezia has been working for Biomathematics & Statistics Scotland since February 2008. His research interests include Bayesian modelling in time and space; Bayesian model choice and variable selection; computational statistics; environmental and ecological statistics. His expertise is in developing temporal and spatial models with a latent Markov process, e.g. hidden Markov models and spatial hidden Markov models for the classification of the observations into a small set of homogeneous groups; Markov switching autoregressive models for the analysis of non-linear and non-normal time series. He applied his models to stochastic hydrology, image analysis, biogeography, animal movements, and, air quality control. His methodological research is currently developed under the module Multivariate time series models for sensor and sensor network data. The list of Luigi’s publications is available at www.bioss.ac.uk/~luigi.
- Alberto Sorrentino (Department of Mathematics, University of Genova)
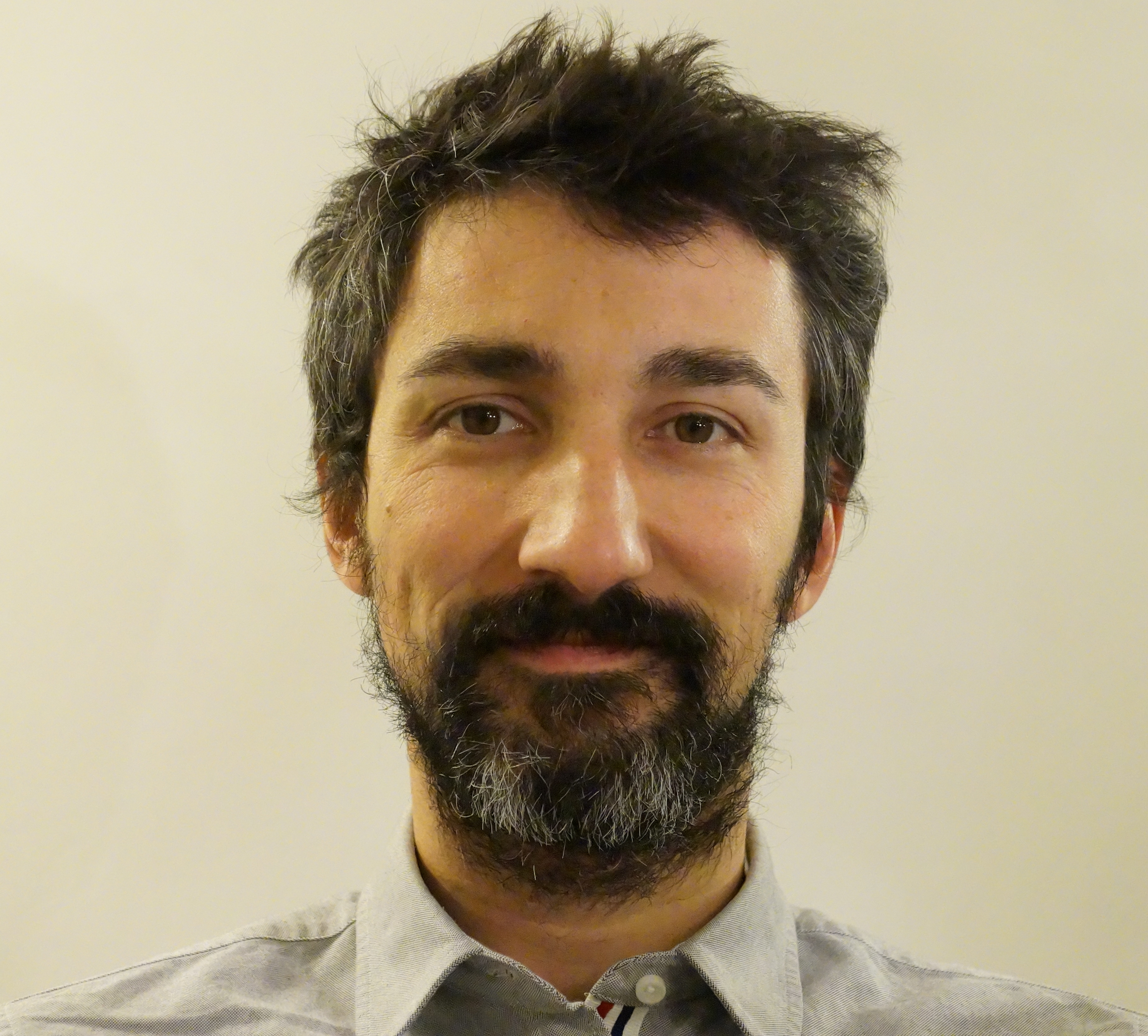
Alberto Sorrentino works is at the boundary between mathematics, statistics and applications. He mostly works in inverse problems, usually within a Bayesian framework, and often use Monte Carlo algorithms for solving non-linear/non-Gaussian problems. As far as applications are concerned, he have worked at methods and algorithms for Electro-/Magneto-encephalography, Magnetic Resonance Imaging, astronomical imaging and LIDAR data analysis. He is currently involved in a couple of projects for improving localization of epileptogenic areas from high-density EEG data.
- Piero Poletti (Fondazione Bruno Kessler, FBK)
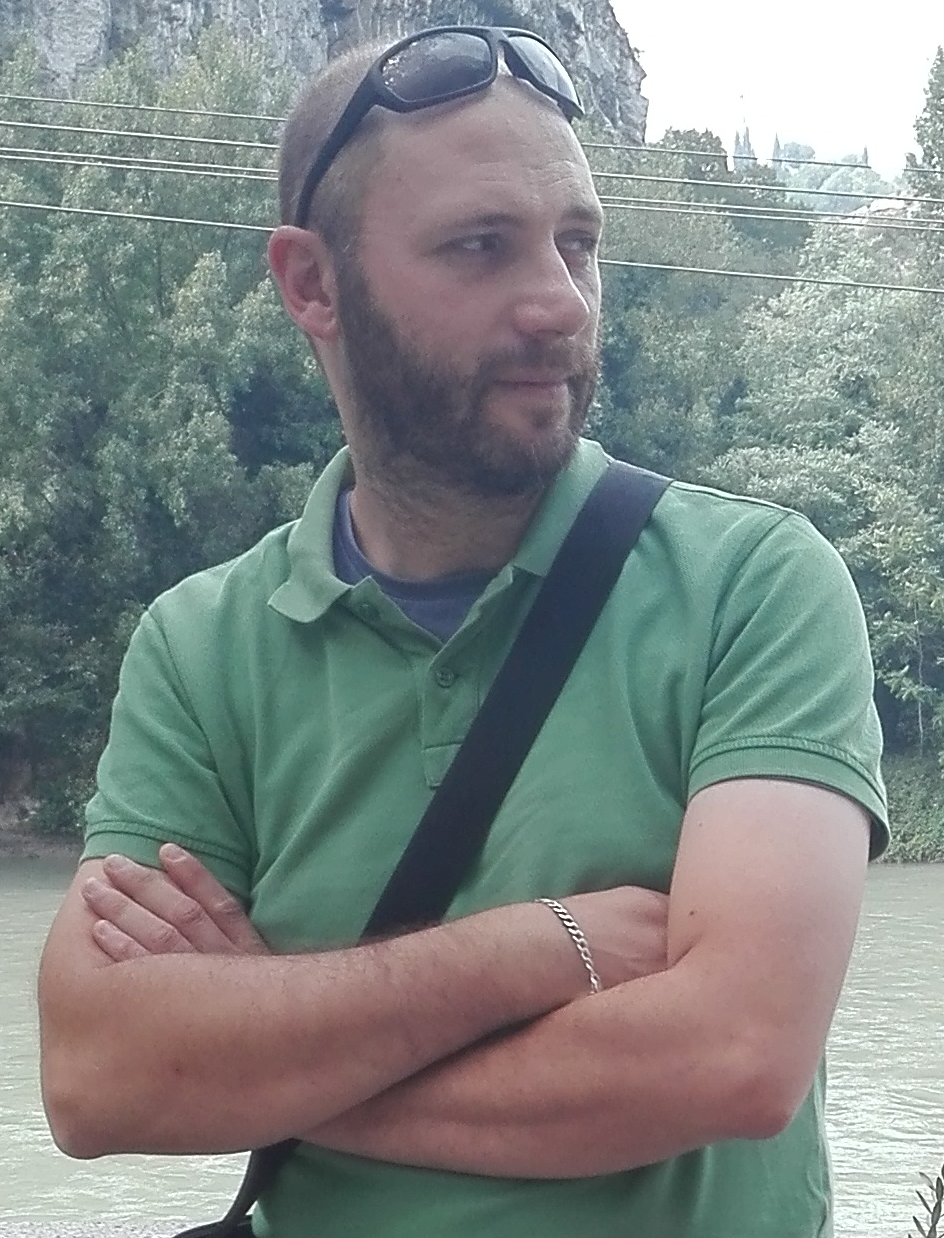
Piero Poletti is research scientist at the Fondazione Bruno Kessler (FBK), within the Dynamical Processes in Complex Societies (DPCS) unit. His research focuses on the development and analysis of computational models for investigating the epidemic spread in human populations and for evaluating the effect of control measures and disease containment/mitigation strategies. His research interests include: large scale simulation of emerging infectious diseases, the evaluation of vaccination policies for childhood diseases, the analysis of how population heterogeneity and demographic changes can affect the epidemiology of childhood diseases, the cost-effectiveness analysis of vector control activities to reduce the spread of mosquito-borne diseases, and the simulation of human behavioural changes in response to the perceived risk of infection.
Program
Day 1 (27/06/2018)
- [08:00- ] Registration Desk is open
- [08:15-8:30] Welcome
Introduction to Bayesian Statistics (Part 1)
Room A107, [8:30-10:00], [10:30-12:30], [14:00-16:00], [16:30-18:00]
Luigi Spezia (Bioss, Scotland)
Modern science is generating large complex data sets which require sophisticated modelling in order to answer questions of scientific interest. Modelling based on the Bayesian paradigm can be the suitable tool to find the answers. During these lectures, Bayesian models, methods, and algorithms for the interpretation of large scale real-world data set will be introduced. The course will cover the following topics: Bayes and his formula; Bayesian inference; Bayesian modelling; Markov chain Monte Carlo; and examples from linear models; generalized linear models; time series models; mixture models; model choice; and variable selection.
References
- Gamerman D and Lopes HF (2006). Markov Chain Monte Carlo: Stochastic Simulation for Bayesian Inference, Second Edition. Chapman & Hall/CRC, Boca Raton.
- Gelman A, Carlin JB, Stern HS, Rubin DB (1995). Bayesian Data Analysis. Chapman & Hall/CRC, Boca Raton.
- Hoff PD (2009). A First Course in Bayesian Statistical Methods. Springer, New York.
- Robert CP (2001). The Bayesian Choice, Second Edition. Springer, New York.
- Robert CP and Casella G (2010). Monte Carlo Statistical Methods, Second Edition. Springer, New York.
Slides (username: PDS2018)
Day 2 (28/06/2018)
Introduction to Bayesian Statistics (Part 2)
Room A107, [8:30-10:00], [10:30-12:30]
Luigi Spezia (Bioss, Scotland)
Modelling Neural Activity by Magneto-/Electro-Encephalography (M/EEG), Part 1
Room A107, [14:00-16:00], [16:30-18:00]
Alberto Sorrentino (University of Genova)
The lectures describe and discuss Bayesian methods for inference of neural activity from non-invasive recordings obtained by magneto-/electro-encephalography (M/EEG).
M/EEG record, with millisecond resolution, the magnetic/electric field produced by the neural electrical activity: from these recordings, one can obtain estimates of the neural currents that have generated them.
Depending on the physical model used to describe the neural currents, the statistical model is either linear or non-linear. In addition, due to the high frequency of the recordings, the inference problem can be faced as a dynamic problem, with a Hidden Markov Model structure; as such, it can be solved by Bayesian filtering techniques, such as Kalman filtering (for the linear/Gaussian case) and particle filtering (for the non-linear case).
These lectures provide a formal description of the M/EEG problem, together with an overview of the applications. It will then go on reviewing how different methods (i.e. different physical models/prior assumptions) impact the estimate of neural activity from M/EEG data. Finally, we will briefly discuss the problem of reconstructing brain networks by estimation of functional connectivity.
References
Hämäläinen M, Hari R, Knuutila J, Lounasmaa O (1993): Magnetoencephalography: Theory, instrumentation and applications to non-invasive studies of the working human brain. Rev Mod Phys 65:413–498.
Somersalo E, Kaipio J (2004): Statistical and Computational Inverse Problems. New York: Springer Verlag.
Slides (username: PDS2018)
Day 3 (29/06/2018)
Modelling Neural Activity by Magneto-/Electro-Encephalography (M/EEG), Part 2
Room A107, [8:30-10:00], [10:30-12:30]
Alberto Sorrentino (University of Genova)
Bayesian methods applied to computational epidemiology
Room A107, [14:00-16:00], [16:30-18:00]
Piero Poletti (Fondazione Bruno Kessler)
Mathematical and computational models, mimicking the key mechanisms of transmission of infectious pathogens, can provide important insights on the epidemiology of infectious diseases. In particular, models and simulations can be used to better understand the temporal and spatial spread of the infection in human populations and to predict the effectiveness and cost-effectiveness of public health control strategies aimed at interrupting the transmission. Routine epidemiological surveillance mainly reports on clinically apparent cases of infection, while key determinants of the disease spread often remain hidden variables of the underlying transmission dynamics. Statistical inference and Bayesian approaches are particularly useful to calibrate epidemiological models and estimate unknown model parameters on the basis of available socio-demographic and epidemiological data. The course will provide an overview of some applications of Bayesian methods to computational epidemiology and it will be structured as follows:
- Introduction to simple mathematical models, based on ordinary differential equations, for investigating the transmission dynamics of infectious diseases.
- Discussion on the level of model complexity required for investigating some illustrative research questions.
- Description and discussion of key ingredients affecting the spread of different infectious diseases in human populations.
- Insights on how model estimates and forecasts can be provided and used during new emerging epidemic threats. Epidemiological applications will range from the analysis of measles outbreaks recently occurred in Italy (2017) and in Ethiopia (2013-2017) to the simulation of the spread of Zika virus in the Americas (2015-16) and Ebola Disease in West Africa (2014-2016).
References
- Zhang Q et al. Spread of Zika virus in the Americas. Proc Natl Acad Sci USA, 2017.
- Trentini F et al. Measles immunity gaps and the progress towards elimination: a multi-country modelling analysis. Lancet ID, 2017.
Slides (username: PDS2018)
Organizers
- Claudio Agostinelli (claudio.agostinelli@unitn.it)
- Andrea Pugliese (andrea.pugliese@unitn.it)
- Alberto Valli (alberto.valli@unitn.it)
Information
In case you need more information you can contact Claudio Agostinelli (claudio.agostinelli@unitn.it).